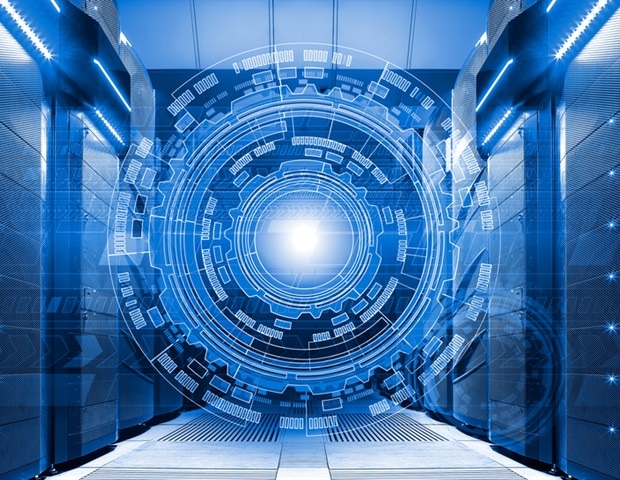
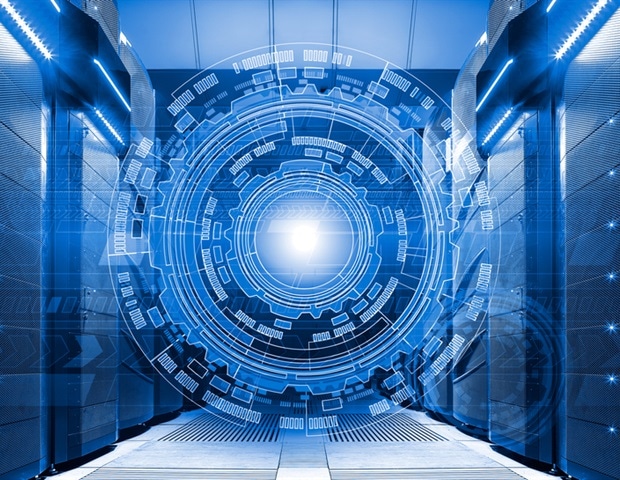
An approach based on artificial intelligence (AI) may allow EKGs to be used to screen for hypertrophic cardiomyopathy in the future. With hypertrophic cardiomyopathy, the heart walls become thick and may interfere with the heart’s ability to function properly. The disease also predisposes some patients to potentially fatal abnormal rhythms. Current EKG technology has limited diagnostic yield for this disease.
New Mayo Clinic research suggests that a convolutional neural network AI can be trained to detect unseen characteristics of hypertrophic cardiomyopathy. The standard 12-lead EKG is a readily available, low-cost test that can be performed in many settings, including those with limited resources.
Hypertrophic cardiomyopathy may be underdiagnosed because it often does not cause symptoms. Patients are often unaware they have it until they experience complications, but early identification can be important. Hypertrophic cardiomyopathy is one of the leading causes of sudden death in adolescents and young adults participating in sports.
Peter Noseworthy, M.D., a Mayo Clinic cardiologist, suggests that AI might offer an effective and readily-available method for earlier diagnosis of hypertrophic cardiomyopathy through an EKG. Dr. Noseworthy is senior author on a newly published study in the Journal of the American College of Cardiology: “Detection of Hypertrophic Cardiomyopathy Using a Convolutional Neural Network-Enabled Electrocardiogram.”
Researchers trained and validated a convolutional neural network using digital 12-lead EKG from 2,448 patients known to have hypertrophic cardiomyopathy and 51,153 who did not, matching the control subjects for age and sex. Next they tested the AI’s ability to detect the disease on a different group of 612 subjects with hypertrophic cardiomyopathy and 12,788 control subjects.
For diagnostic tests such as this neural network, the diagnostic performance is measured mathematically through the area under the receiver operating characteristic curve, on a scale where 0.5 is poor (flip of a coin) and 1.0 is excellent (perfect test). The measurement relates to the test’s ability to correctly identify patients who have the disease (sensitivity), and correctly identify patients who do not have the disease (specificity).
For comparison, a typical positive Pap smear test would have an area under the curve of 0.7 and a mammogram would be 0.85. The study found the AI’s ability to determine patients with hypertrophic cardiomyopathy from those without it had an area under the curve of 0.96 – a powerful predictor.
The good performance in patients with a normal EKG is fascinating. It’s interesting to see that even a normal EKG can look abnormal to a convolutional neural network. This supports the concept that these networks find patterns that are hiding in plain sight.”
Dr. Peter Noseworthy, M.D., Mayo Clinic cardiologist
The study also tested the AI on subgroups. The area under the curve for predicting hypertrophic cardiomyopathy in a group of patients diagnosed with left ventricular hypertrophy, a disease caused by high blood pressure that also is characterized by heart wall thickening, was 0.95. The area under the curve in the subgroup with only normal EKGs was also 0.95. The area under the curve for the subgroup of patients diagnosed with aortic stenosis (narrowing of the valve) was 0.94. The test performed similarly well in a subset of patients who had been genetically tested and confirmed to have pathogenic mutations for the disease.
“The subgroups are important for understanding how to apply the test. It’s good to see that the AI performs well when the EKG is normal as well as when it is abnormal due to left ventricular hypertrophy,” says Konstantinos Siontis, M.D., a resident cardiologist at Mayo Clinic and co-first author of the study. “Perhaps even more important is the fact that the algorithm performed best in the younger subset of patients in our study (under 40 years old), which highlights its potential value in screening younger adults.”
More research remains to be done, such as testing the AI in other adult populations, children and adolescents to find out where it works well and where it fails.
“This is a promising proof of concept, but I would caution that, despite its powerful performance, any screening test for a relatively uncommon condition is destined to have high false positive rates and low positive predictive value in a general population. We still need to better understand which particular populations will benefit from this test as a screening tool,” says Dr. Siontis.
“We also need to learn more about what specific characteristics of hypertrophic cardiomyopathy this network is detecting. We hope to learn how to apply this technology to screening and managing patients in families affected by this disease,” adds Dr. Noseworthy.
Ko, W-Y., et al. (2020) Detection of Hypertrophic Cardiomyopathy Using a Convolutional Neural Network-Enabled Electrocardiogram. Journal of the American College of Cardiology. doi.org/10.1016/j.jacc.2019.12.030.